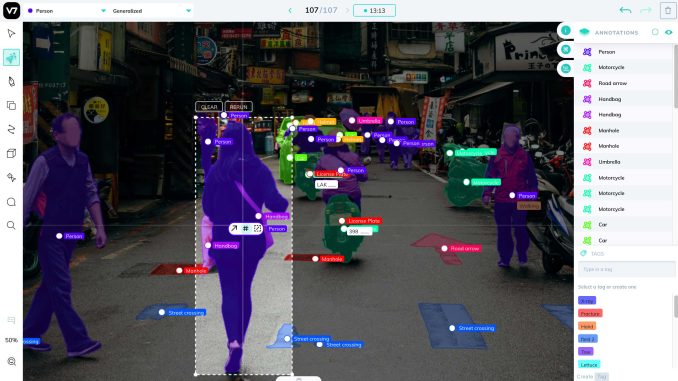
Annotation enable individuals to engage texts interactively through making notes, highlighting words and phrases, and marking up selections of text that increase comprehension, retrieval, and reaction to what is read by a user, an audience, machine or a character. It is characterized explicitly as grappling with a piece of writing through pointing out important details, conceptualizing main themes, and listing queries or reflections.
Annotation is a crucial process in various fields, including machine learning, computer vision, and natural language processing. Data annotation helps readers:
How Artificial Intelligence Is Revolutionizing the Insurance Industry
- Understand and Retain Information: It aids in understanding and keeping the information longer by engaging and making summaries of the text.
- Organize and Structure: It helps to isolate and organize important material, identify key concepts, and monitor learning as you read.
- Prepare for Exams and Discussions: Annotated notes may simplify test preparations, as well as promote conversations by offering a comprehensive summary in a straightforward manner.
- Identify the most relevant and reliable sources.
- Understand the strengths and limitations of each source.
- It analyzes varied sources and studies relationships in depth to understand the topic more extensively.
Types of Annotation
1. Video Annotation
Video annotation is vital in teaching machines how to see, especially for artificial intelligence. In essence, it entails marking video frames using various segmental properties.It involves the following steps:
- Importing or uploading files into an annotation platform
- Creation of a new project and adding the file above
- Annotating keypoint frames: The keypoint frames are annotated using chosen tools and markup labels such as cuboids, bounding boxes, skeletal, skeleton, lines, points, bitmap, semantic segmentation, etc.
- Exporting annotations: There are different formats in which the annotated frames are exported such as JSON and XML which can be used in machine learning projects.
Best practices for video annotation include:
- Ensuring clear guidelines and training materials for annotators.
- Maintaining quality control by manually checking each markup and ensuring consistency across frames.
- Tracking changes made by different users.
- Using classification models to create new labels.
- Iterating over time to improve the quality of annotations.
2. Text Annotation
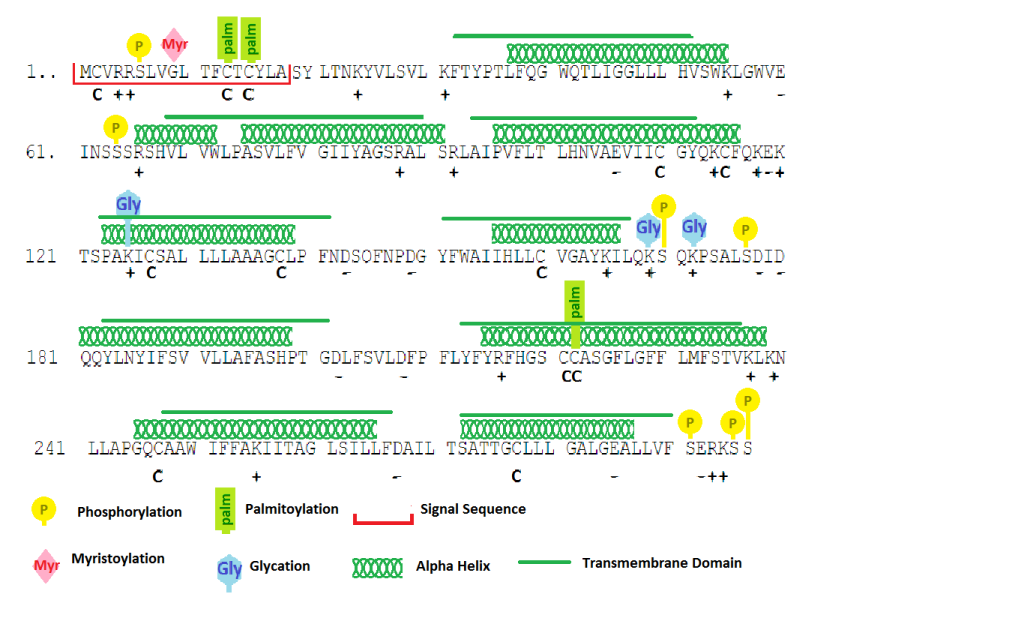
It is the process of adding value to a bunch of words by identifying and defining different parts such as definitions, meaning, and purpose is referred to as text annotation. Whenever it comes to natural language processing (NLP) and machine learning, this is vital. Techniques touched on text annotation encompass:
- Named Entity Tagging: Locating and tagging special purposes in text (i.e., locations, individual names, businesses).
- Sentiment Annotation: Assigning sentiment based labels including; negative and neutral –positive extreme sentiments if any in text.
- Semantic Annotation: Labeling text with domain-specific information or intent (e.g., sarcasm, irony).
The Best Practices for Text Annotation include:
- Collecting multiple annotations on the same text to ensure accuracy.
- Using inter-annotator agreements to determine correct annotations.
- Utilizing gold standard datasets as references.
- Crowdsourcing text annotation for large-scale projects.
3. Image Annotation
It is a process of designating pictures with annotation information and attributes which allow computers to better understand objects. Techniques involved in image annotation include;
- Bounding Boxes: Drawing boxes around objects to attribute object-specific details.
- Landmarking: Identifying specific points or features on an object.
- Polygons: Drawing polygons to define regions within an image.
- Object Detection: Identifying and labeling specific objects within an image.
The Best Practices for Image Annotation include:
Through utilization of image annotation tools that have specific characteristics as well as functionalities.
By executing them under the guidance of an image annotation expert, we can guarantee highest possible quality annotations.
Utilizing various annotation techniques based on project requirements.
4. Annotation Bibliography
An annotated bibliography is essentially a list of sources that were used for gathering information on a particular topic. It is made up of small descriptions or evaluations about each of them. Consequently, an annotated bibliography is able to present information on consulted sources, demonstrating their appropriateness, authenticity and credibility.
This form of annotation is essential for learning about a topic, formulating a thesis, and helping other researchers understand a topic.
Techniques for Annotating
Define a format: Establish a consistent format for your annotations, such as using parentheses for names or circles for unknown words.
Summarize Key Points: Key points of the text summarized in your own words using headers and bold text for reference.
Circle Key Concepts: You can find important words and phrases by circling them, or you might want to check what an unfamiliar term means.
Write Brief Comments and Questions: Insert some quick comments and questions on the side-lines. This will help you connect with what you read and spring your thoughts into action.
Use Abbreviations and Symbols: Design a system of abbreviations and symbols to identify significant points or questions quickly.
Highlight and Underline: Highlight or underline key points, but do it in a way that is not excessive.
Sticking to a System: Consistency is key to making annotations effective and easy to review.
Use Digital Tools: Use items such as PDF annotation apps, the web-based textbooks or browser extensions in making annotations on your text.
Keep It Simple: Avoid overwhelming the text with too much information and focus on a specific purpose for your annotations.
Make it Aesthetic: To make your annotations visually appealing use number of cool book annotation distribution items like sticky notes, tabs, and colorful pens.
Data Annotation Jobs in the USA
There are many jobs for data labeling in America. Here are some of the top platforms and companies where you can work as a data labeling officer.
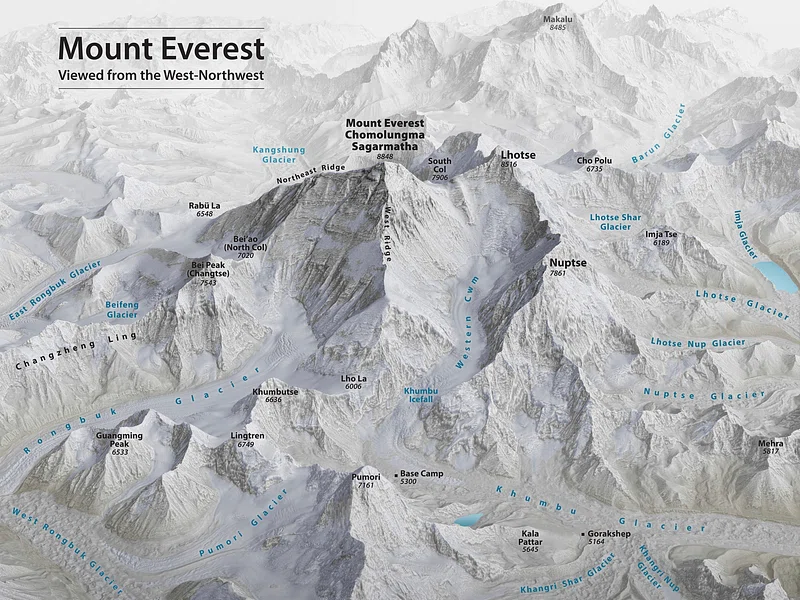
1. Triyock BPO:
Triyock offers specialist services in data labeling and annotation such as data labeling, image and video annotation and text annotation meant for machine learning and AI models.Therefore, the company gives high-quality annotation services for different sectors this includes such as e-commerce, retail, healthcare industries.
2. Kili Technology:
Kili Technology is a tool for marking that professionally marks images, videos, PDFs and texts. Its workflows are strong and there are modern measures of quality to ensure that data is quickly and accurately marked.
3. Remote Labeler:
The Remote Labeler is a company that specializes in data annotation offering a wide array of services which include image annotation, video annotation, text annotation, speech annotation and so on. They ensure high quality and accurate data annotation by use of sophisticated annotation tools and technologies.
4. iMerit:
iMerit is a company that labels data well for AI uses through computer vision, human judgment or language processing . They provide many labeling services such as image annotation videos annotating and text tagging.
5. Upwork:
Offering different data annotation works such as Image Annotation, Text Annotation, among others, Upwork platform is great for freelancers specializing in that line. On this platform, you can get these roles anywhere worldwide and for any level of expertise as well as time.
6. Encord:
Encord is a smart development tool that handles AI data and it offers advanced annotation features that are good for computer vision applications. It also consolidates and automates the steps of curating, annotating, and validating data which are very important for companies that need large scale high performance image recognition systems.